Prof. Ing. Peter TREBUŇA, PhD.
Bio:
(*1980) graduated from the Faculty of Mechanical Engineering at Technical University in Košice in the field of study Economics and Management of Mechanical Engineering (2003) on the Faculty of Economics of the Technical University in Košice in Finance, Banking and Investment (2003). In 2006 he passed his PhD thesis on “Methodology of business culture” in the scientific area “Business Management” at the Slovak University of Technology in Bratislava on the Faculty of Materials Science and Technology in Trnava. In 2010 he habilitated as the associated professor with the theme “Application of experimental modelling methods in industrial engineering” in the scientific field “Industrial Engineering” at the Faculty of Mechanical Engineering in Košice. In 2016 he was inaugurated as a professor with a theme of Production simulation using the digital twin concept.
Currently, he is a professor at the Department of Industrial and Digital Engineering of the Faculty of Mechanical Engineering at the Technical University in Košice. He is also a garant of several subjects in the field of study: Industrial Engineering at the 1st, 2nd and 3rd level. During 2005-2023, he participated in the lecture stay within the Erasmus teacher mobility project at the Institute of Economics and Management, Faculty of Mechanical Engineering, Czech Technical University in Prague, University of West Bohemia, University of Zielona Gora Poland. He is the co-author of 7 monographs, 10 university textbooks, 9 scripts and the results of his research activities were published in more than 500 domestic and foreign journals and at conferences at home and abroad. He cooperated and still cooperated on solving practical problems (more than 30). He also participated in the solution: the role of the state plan of research and development, grant projects VEGA, KEGA and Applied research APVV. As the principal solutionist, resp. The deputy of principal solutionist has completed 3 projects VEGA, 2 projects KEGA and 1 APVV. Currently, he is the leader of project VEGA and project KEGA solved at the domestic department. Professor Trebuňa is a member of the Scientific Board of FME TUKE, FST TNUNI, a member of ZAPSR, member of scientific committees at conferences and journals.
Prof. Ing. Jānis Grabis, PhD.
Bio:
Jānis Grabis is a Professor at the Faculty of Computer Science and Information Technology, Riga Technical University, Latvia. He obtained his PhD. from the Riga Technical University in 2001. He worked as a Research Associate at the College of Engineering and Computer Science, University of Michigan-Dearborn, and Stockholm University. His research interests are in enterprise integration, simulation and supply chain management. He has published in major academic journals, including OMEGA, the European Journal of Operational Management, the International Journal of Production Research, Computers & Industrial Engineering, Software and Systems Modeling and others. He has been a guest editor for four top academic journals and a chair of the program committee of several international academic conferences. Jānis Grabis has co-authored a monograph on supply chain configuration. He collaborates extensively with industry, including companies such as SAP, Ford Motor Company, TET and ZZ Dats.
Abstract:
Supply chains are complex networks subject to internal and external disruptive events. Resilience is one of the most important properties of modern supply chains.
As part of the lecture, you will receive information about comprehensive methods that should be implemented into continuous monitoring, analysis and automated control of supply chains to achieve resilience. The proposed research describes ways of integrating descriptive, prescriptive and predictive models to meet these requirements. Hybrid simulation-optimization models are a part of the presented research.
Optimizing, in this case, allows the evaluation of a number of supply chain designs, and simulation accounts for dynamic and stochastic aspects. The hybrid model combines the strengths of both methods and is developed based on a standard conceptual supply chain model. From the lecture, you can also get information about the overall modelling approach, theoretical aspects of hybrid modelling, and practical examples of supply chain modelling.
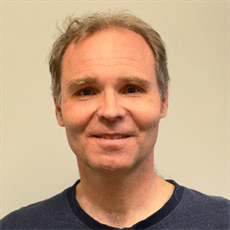
Prof. Peter Tiňo
Title: Recurrent Neural Networks – Past, Present, Future
Bio:
Professor Peter Tiňo holds a Chair position in Complex and Adaptive Systems at the School of Computer Science, University of Birmingham, UK. His interests span machine learning, neural computation, probabilistic modelling and dynamical systems. Peter is fascinated by the possibilities of cross-disciplinary blending of machine learning, mathematical modelling and domain knowledge in a variety of scientific disciplines ranging from astrophysics to bio-medical sciences.
He has served on editorial boards of a variety of journals including IEEE Transactions on Neural Networks and Learning Systems, IEEE Transactions on Cybernetics, Scientific Reports, and Neural Computation and (co-)chaired Task Force on Mining Complex Astronomical Data and Neural Networks Technical Committee (TC of IEEE Computational Intelligence Society). Peter led an EPSRC-funded consortium of six UK universities on developing new mathematics for personalised healthcare.
He was a recipient of the Fulbright Fellowship to work at NEC Research Institute, Princeton, USA, on dynamics of recurrent neural networks, UK–Hong-Kong Fellowship for Excellence and three Outstanding Paper of the Year Awards from the IEEE Transactions on Neural Networks and the IEEE Transactions on Evolutionary Computation.
Abstract:
An introduction of a “neural memory” in RNN seems quite straightforward, and we are still far from fully understanding their workings. For a better understanding of this area of research, I will present my personal view of the developments in this field with an emphasis on key ideas that helped to push our understanding forward.
When learning from “dynamic” data where the order in which the data is presented matters, the key issue is how such temporal dependencies get captured within the learning machine.
In the case of artificial neural networks, an often adopted strategy is to introduce feedback-connections with time delay(s). This enables the neurons to form their activation patterns based on past as well as current neural activations. Neural networks of this kind are known as Recurrent Neural Networks (RNN).
Many diverse architectures fall under the umbrella of RNN (from simple RNN to LSTM and Transformers), with application domains ranging from language processing and narrative production to bioinformatics and chaotic time series modelling and prediction.
In the final part of my presentation, you will get information about my vision and speculate about possible directions in which this field will be explored in the future and be able to participate in the discussion.